Construction of Statistical Shape Models from Minimal Deformations
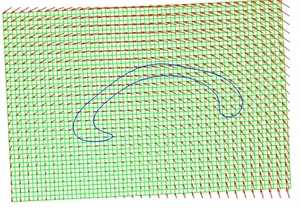
Please use this identifier to cite or link to this publication: http://hdl.handle.net/10380/1503
New: Prefer using the following doi: https://doi.org/10.54294/eoxxuh
Published in The MIDAS Journal - MICCAI 2008 Workshop: Manifolds in Medical Imaging: Metrics, Learning and Beyond.
Abstract. Statistical shape models (SSM) capture the variation of shape across a population, in order to allow further analysis. Previous work demonstrates that deformation fields contain global transformation components, even if global pre- registration is performed. It is crucial to construction of SSMs to remove these global transformation components from the local deformations - thus obtaining minimal deformations - prior to using these as input for SSM construction. In medical image processing, parameterized SSMs based on control points of free-form deformations (FFD) are a popular choice, since they offer several advantages compared to SSMs based on dense deformation fields. In this work, we extend the previous approach by presenting a framework for construction of both, unparameterized and FFD-based SSMs from minimal deformations. The core of the method is computation of minimal deformations by extraction of the linear part from the original dense deformations. For FFD-based SSMs, the FFD-parameterization of the minimal deformations is performed by projection onto the space of FFDs. Both steps are computed by close-form solutions optimally in the least-square sense. The proposed method is evaluated on a data set of 62 MR images of the corpus callosum. The results show a significant improvement achieved by the proposed method for SSMs built on dense fields, as well as on FFD-based SSMs.